Navigating Ethics and Equity in the Pursuit of Responsible AI
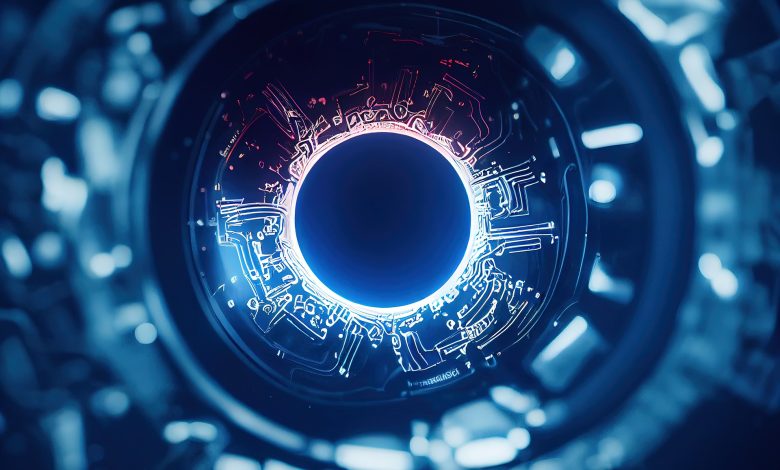
By Srini Koushik, President of AI, Technology and Sustainability and CTO at Rackspace
Since OpenAI’s release of ChatGPT to the mainstream consumer market, the adoption of generative AI technologies has been astounding. Yet, while the use of other foundational models – covering language, code, and visuals – has accelerated innovation, it has also resulted in misuse.
As AI can process large volumes of personal data, there are concerns that AI can be used maliciously in cyber-attacks, enabling more effective phishing attempts or bypassing security measures.
Some organisations have responded to this with outright bans. But besides potentially missing out on the transformative power of generative AI, this drastic measure is also not very effective. Deloitte, for example, finds that nearly half of employees across the Asia-Pacific are using this technology to do their jobs better and faster.
Defining responsible AI
Unlike accountability, responsibility isn’t purely about individual or co-ownership – but rather collective ownership and authentic alignment between words and deeds. In the AI context, this means ensuring developers and users consider potential impacts on individuals and society.
Responsible AI, then, hinges on focusing on the underlying processes. AI shouldn’t merely just drive innovation or improve productivity. Instead, organisations must collectively ask how that is being done. Is it ethical, trustworthy, fair unbiased, transparent, and beneficial to individuals and society as a whole? In other words, it is about using AI as a decision-support system and not as a decision-maker.
Forging comprehensive trust
With AI systems intrinsically having multiple information layers encoded in deployed models, it can be hard to clarify what is shared. Typically, foundational models are available from a variety of sources, ranging from proprietary to open source. These are then expanded and incorporated into various functional models, which are further incorporated into products.
Consider GitHub Copilot, for instance. Developers using Copilot for AI pair programming need to be aware of the proprietary IP and data shared with the platform while co-creating code. The first level of this trust chain lies with individual users. Organisations should establish policies and governance regarding the use of GitHub Copilot, which represents the second layer of trust in the software product.
GitHub, in turn, relies on OpenAI Codex as the foundational model, thereby placing a further level of trust in OpenAI. Responsible use, therefore, hinges on understanding what data GitHub collects as well as what OpenAI gathers. This creates a necessary chain of trust: GitHub must trust that OpenAI acts ethically, while we, in turn, must trust GitHub and our colleagues to use the service responsibly.
The approach to responsible AI
Decision-makers today are striving to build resilience in their organisations, and this includes accounting for the disruption from generative AI. PwC finds that in the Asia-Pacific, almost half of CEOs surveyed were concerned about cybersecurity risks from this technology. Meanwhile, 44% said they were worried about generative AI being used to spread misinformation. This doesn’t detract from the fact that generative AI can be used to build an intelligent enterprise with the use of secure, affordable, and scalable AI models.
Here are some guidelines taken in mitigating steps to make policies that are applicable, actionable, and tied to our use case scenarios:
- Keep policies simple and easy to understand.
- Define data classification policies and provide guidance that includes concrete examples of information classification and the secure use of data.
- Educate and empower teams with responsible AI principles and guidelines, and contextualise the policies with real-world examples.
- Implement a process for monitoring the ethical usage of AI.
- Create a governance council that can triage and validate the application of policies and make regular updates to them.
Core AI policies
- Governance and oversight: Forming a committee and defining owners to provide oversight, compliance, auditing, and enforcement of the AI standard.
- Authorised software use: Use is subject to the same global purchasing and internal use oversight that is applied to other software applications.
- Responsible and ethical use: Encourage ethical use, supervision and explainability of AI models by ensuring validity, reliability, safety, accountability, transparency, our ability to explain and interpret fairness, and the management of harmful bias.
- Confidential and sensitive information: Implemented information classification standards and provided clear guidance on the usage of AI services to ensure the proper protection of intellectual property, regulated data, and confidential information.
- Data retention, privacy, and security: Uphold data management and retention policies and maintain compliance with corporate security and data privacy policies.
- Reporting: Encourage reporting of violations of the AI standard in good faith.
Here are some core guiding principles to help create policies and build a socially responsible environment, and this includes:
- AI for good – Create and use AI for the collective good and limit harmful factors.
- Eliminate bias – Be fair and remove bias through algorithms, datasets, and reinforcement learning.
- Accountable and explainable – Hold ourselves accountable for any use of AI and derivative uses and employ explainability as a foundation for any model-building process.
- Privacy and IP – Maintain a secure use of corporate data and intellectual property.
- Transparency – The use of models and data sets are well catalogued and documented.
- Ethical use – Monitor and validate the ethical use of datasets and AI.
- Improved productivity – Focus efforts on AI adoption to improve productivity, increase operational efficiencies, and spur innovation.
Ethical AI encourages fair outcomes for all users and aids in preventing unexpected repercussions like bias or discrimination. Businesses must adopt a strategy that combines supervision and governance to guarantee that AI-driven innovations continue to be reliable and consistent with societal norms.